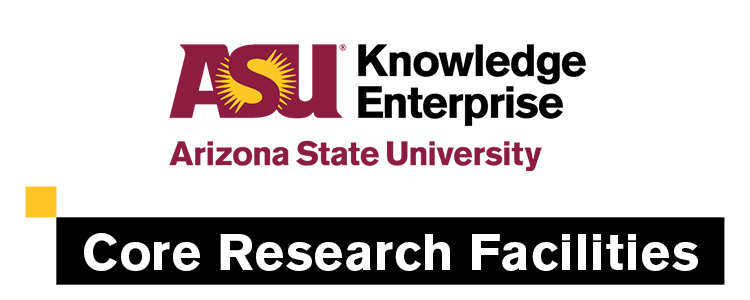
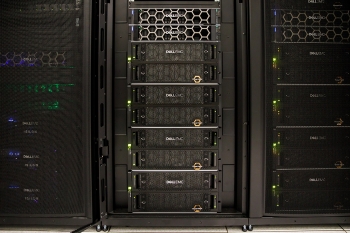
Welcome to the ASU Core Facilities Newsletter. We are ready to support all your research goals. Please follow our LinkedIn page for additional resources and community information.
Highlight: Research Computing
As a vital resource at Arizona State University, Research Computing is dedicated to advancing research, fostering innovation and driving discovery. With a team of seasoned professionals, they provide expertise in parallel computing, big data analytics, scientific visualization, high-speed networking and cybersecurity.
For ASU researchers, Research Computing is the key to unlocking the potential of advanced computational resources, enabling you to pursue groundbreaking work in science, engineering and health.
News
ASU Knowledge Enterprise Vice President and Chief Scientific and Technology Officer Neal Woodbury Demos Large Language Models
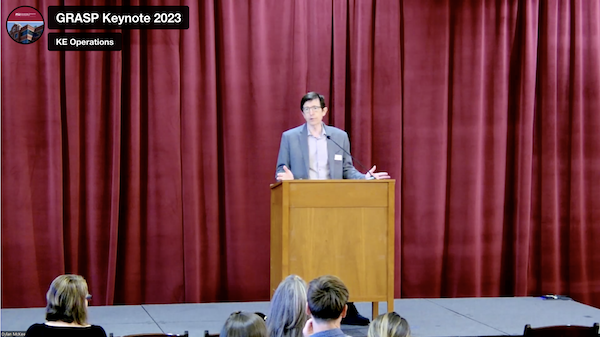
Large Language Models (LLMs) are a form of generative AI, providing responses to input text queries based on billions of parameters.
Using open-source AI models downloaded to local platforms offers several advantages. It removes the necessity to upload intellectual property to proprietary cloud models. Additionally, it allows for greater flexibility and precision in how LLMs are utilized.
LLM interfaces can search datasets they weren't trained on and can even provide answers with source citations. ASU Research Computing offers easy-to-use interfaces and the capability to allocate hardware for brief interactions with advanced LLMs. For extended use, users can be supported by resources within Enterprise Technology.
This project was enabled by the Top 500-ranked ASU Research Computing Sol Supercomputer
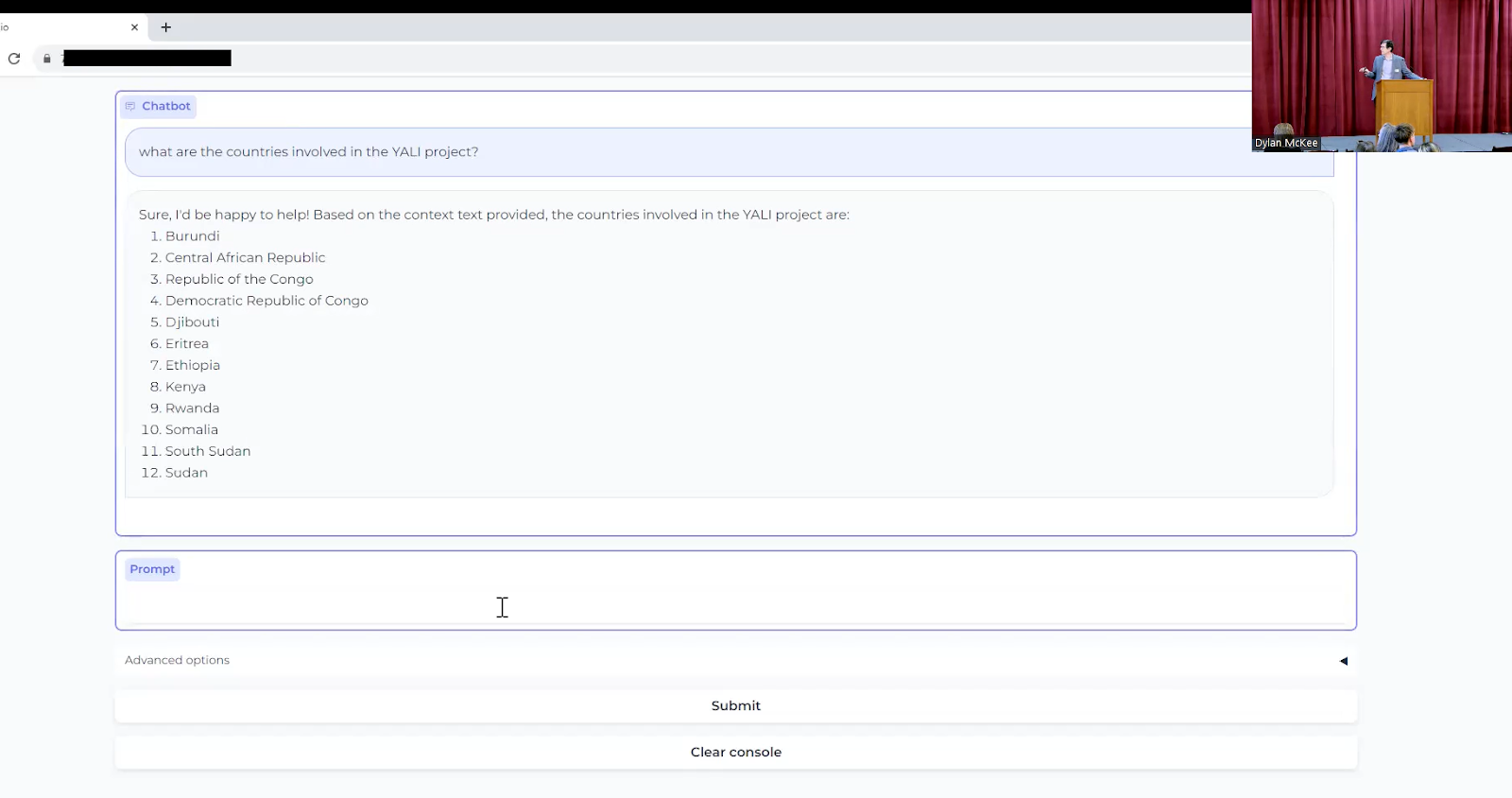
At GRASP 2023, Neal Woodbury showcased the LLM being developed at ASU to the university's research community. Using a straightforward chat interface, he searched a local text collection consisting of over a thousand PDF pages related to the .
To learn more about Sol or to learn more about LLM support with ASU Research Computing visit researchcomputing.asu.edu or schedule a consultation or demo of these LLMs by visiting https://rto.asu.edu/request-help.
Publications
The Sol Supercomputer at Arizona State University
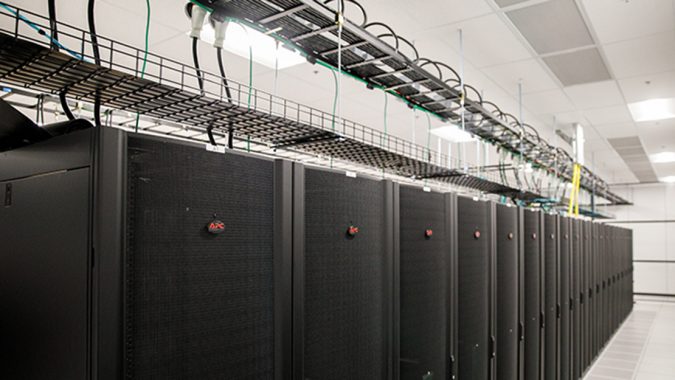
The Sol supercomputer offers ASU researchers cutting-edge capabilities. This paper outlines its rationale and technical aspects, aiming to inspire similar publications at other institutions. The accompanying git repository provides detailed system configurations and can be updated as the system progresses.
Brainomaly: Unsupervised Neurologic Disease Detection Utilizing Unannotated T1-weighted Brain MR Images
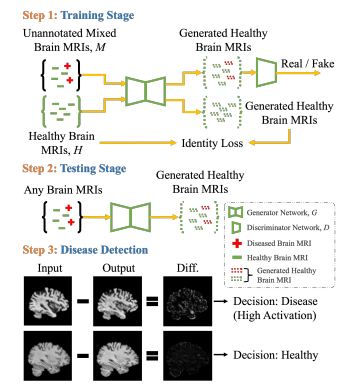
Abstract
Deep neural networks are promising for medical imaging, but obtaining large annotated datasets, especially for rare diseases, is both time-consuming and costly. Anomaly detection methods can help by focusing on images of healthy subjects, but many real-world datasets also include unannotated images of diseased subjects.
Method
We address this with Brainomaly, a method tailored for neurologic disease detection using GAN-based image-to-image translation. Brainomaly specifically caters to neuroimages and optimally uses unannotated mixed datasets. In tests, Brainomaly significantly surpassed other unsupervised methods in detecting Alzheimer’s disease and headaches.
Results
In summary, Brainomaly excels in detecting Alzheimer’s disease and headaches from T1-weighted brain MRIs, notably surpassing current leading methods. Its success is due to its unique image translation approach, ability to utilize unannotated mixed MRIs, and the new AUCp metric for optimal model selection.
Authors: Md Mahfuzur Rahman Siddiquee, Jay Shah, Teresa Wu, Catherine Chong, Todd J. Schwedt, Gina Dumkrieger, Simona Nikolova and Baoxin Li
Read the full research article.